Dipartimento di Filosofia “Piero Martinetti”, Università degli Studi di Milano
04 April 2024
Sala Martinetti
- 14:00-15:00 Juan Durán – TU DELFT
What can philosophy of science and technology teach us about AI?
This lecture serves as an introduction to a range of issues related to the current utilization of AI technology in scientific settings and what insights the philosophy of science and technology can offer in this regard. Some of the topics discussed here derive from popular discussions in the field, such as explanatory AI and the causality/correlation divide. Others await advocates to articulate and present a coherent perspective. Among these are concerns regarding the justification of beliefs, the notion of evidence, and the scientific/technological knowledge distinction. Additionally, the lecture will initiate discussions on topics that lie on the margins of the philosophy of AI but, in the view of this lecturer, deserve greater attention. These include the use of AI for pseudo-scientific endeavors and the future of scientific practice within the realm of AI.
- 15:00-16:00 Silvia Larghi and Edoardo Datteri – RobotiCSS UNIMIB
Mentalistic stances towards AI systems
AI systems, from computer-based conversational agents to embodied robots, may exhibit characteristics that, in some cases, would lead people to attribute them mental states and cognitive abilities (De Graaf & Malle, 2017). Although the literature on the attribution of mental states and cognition to AI systems is extensive and growing (Thellmann, 2022), research on this topic has mainly focused on whether people take an intentional stance (à la Dennett, 1971) towards artificial systems, attributing beliefs, desires and other propositional attitudes to the system to explain and predict its behavior. We suggest that people, in their interaction with AI systems, may occasionally adopt a mentalistic explanatory and predictive strategy, interestingly different from the intentional stance, which involves the decomposition of the system into functional modules processing representations. While the first approach (in Dennett’s framework, the adoption of the intentional stance) is more akin to folk-psychology, the latter, dubbed here folk-cognitivist, is closer to classical cognitivist modeling. The distinction between the two strategies will be explored and illustrated with reference to examples from ongoing empirical researches on the topic. This distinction aims to contribute to our understanding of the dynamic of human-AI interaction which may be relevant to various research fields, to the design of effective, safe and trustworthy interactive AI systems, to the proper dealing with ethical, legal and social issues related to their use, to their deployment as scientific tools to understand human cognition (Wykowska, 2021, Ziemke, 2020).
References- De Graaf, M. M., & Malle, B. F. (2017, October). How people explain action (and autonomous intelligent systems should too). In 2017 AAAI Fall Symposium Series.
- Dennett, D. C. (1971). Intentional systems. The journal of philosophy, 68(4), 87-106.
- Thellman, S., de Graaf, M., & Ziemke, T. (2022). Mental state attribution to robots: A systematic review of conceptions, methods, and findings. ACM Transactions on Human-Robot Interaction (THRI), 11(4), 1-51.
- Wykowska, A. (2021). Robots as mirrors of the human mind. Current Directions in Psychological Science, 30(1), 34-40.
- Ziemke, T. (2020). Understanding robots. Science Robotics, 5(46), eabe2987
- 16:00-17:00 Chiara Manganini and Giuseppe Primiero – PhilTech UNIMI
Defining Formal Validity Criteria for Machine Learning Models
Validity criteria for traditional deterministic computational systems have been spelled out in terms of accuracy, precision, calibration, verification and validation. In the context of scientific simulations, related formal validity requirements have been defined for the relation between the mathematical model underlying the target system and the computational model used for simulating it. With machine learning models entering the picture, these considerations need reviewing, since the conditions under which this relation can be analyzed have largely changed. This is due to a number of reasons: the target system is no longer an available system object of investigation; the mathematical model is still abstracted from the behavior of the system, but is not always given beforehand; the computational model is stable only after the training and the evaluation on the test data set; finally both the learning model and the trained model often remain opaque. Thus, while a classification may result loosely isomorphic to a given trained machine learning model, the latter’s ability to correctly represent reality — and thus to be worthy of being considered epistemologically valid – is still debated. We argue that the underlying relations establishing validity criteria for machine learning models need to be reconsidered in terms of formal relations of probabilistic simulation, and that validation and verification processes for relevant stochastic properties are necessary to this aim.
- 17:00-18:00 Stefano Canali and Viola Schiaffonati – META POLIMI
Emerging Trade-Offs from Personalisation: Health-Related Internet of Things, Wearables, and Medical ML.
In the health context, among the significant promises of Machine Learning (ML) is the prospect of designing systems that can learn on the basis of personal data and thus personalise disease detection and intervention. Personalisation is at the center of scientific and political frameworks such as precision and personalised medicine and is usually connected to various positive changes from epistemological, ethical, and social points of view. However, in this paper we argue that personalisation can exacerbate concerns in the ethics of ML and lead to new trade-offs. Discussing a case study of current uses of ML in relation to wearable and Health-related Internet of Things (H-IoT) devices for health, we show new issues connected to data anonymisation and consent, control and sovereignty, and integration emerge. These results have serious implications for strategies of mitigation for the ethics of ML in health and beyond.
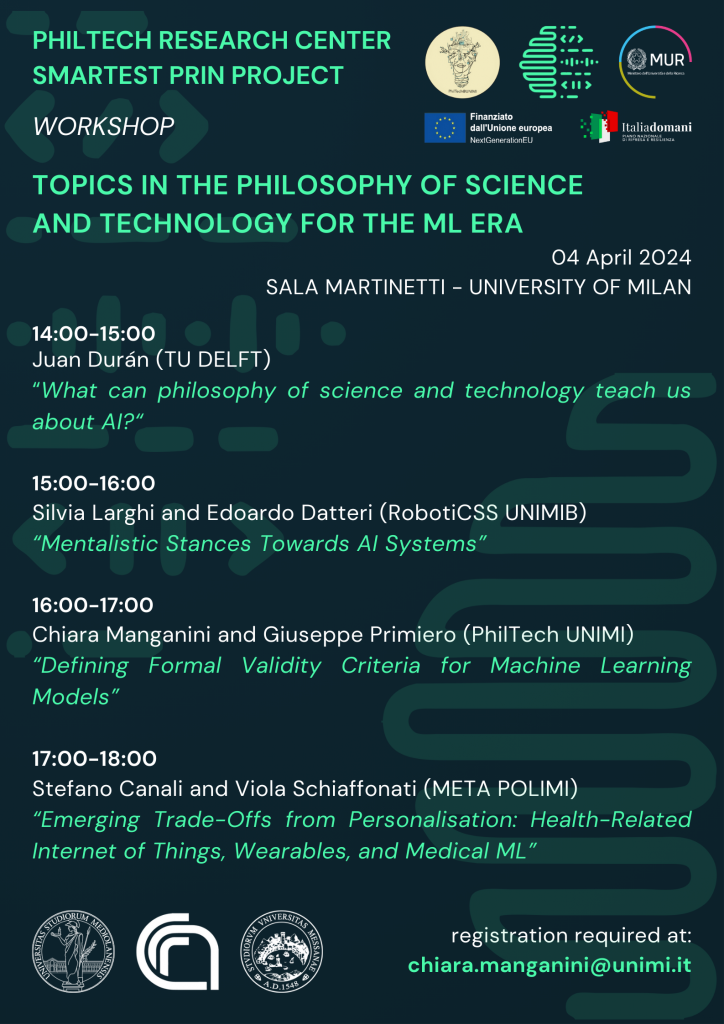